Privacy and security is at Apheris’ core
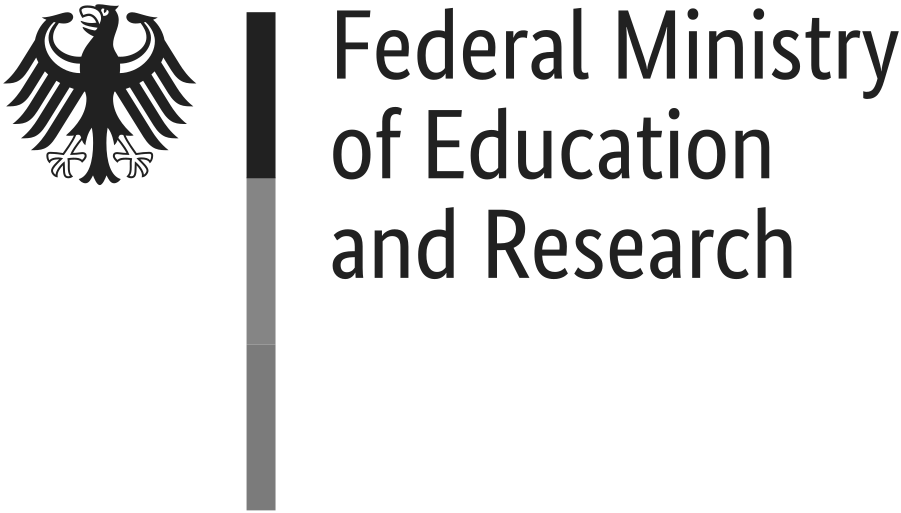
Improve accuracy of your models for your context and processes
Data never moves
Bring algorithms to the data. Allow models to be trained on real world data from financial institutes.
Computational governance
Each financial institute stays in control of their data, approving compute jobs at the computational level. Data that is made available gets updated and can be used to re-train or update models easily.
Seamless federation
Leverage insights from multiple financial institutes with zero code-port.
Insights shared
Choose from multiple privacy-enhancing technologies and ensure only privacy-preserving results are shared.
Access to more real-world transaction data, while remaining compliant
Train models on siloed real world data that can’t be shared due to regulation and compliance requirements. Computational access to data from any bank or financial institute. No data sharing. Remain compliant with both data privacy and industry regulations.
Apheris enables financial institutions and solution providers to collaborate to better fight fraud and money laundering, without transferring or exposing to others any of the raw data or contribution to the collective result.
Apheris enables financial institutions and solution providers to collaborate to better fight fraud and money laundering, without transferring or exposing to others any of the raw data or contribution to the collective result.
Higher accuracy
Adaptive models benefit from continuous monitoring and adaptation. Stay ahead of new money-laundering risks.
Streamlined workflows
Compliance teams focus on high-risk cases and reduce costs associated with false positives.
Proactive detection
Automatically detect behavior that humans might miss.
Scale
Retrain models on newly available data and deploy seamlessly across new sites.
Compliant, private, secure
Data remains with the financial institute reducing the risks that come with sharing or centralizing data. Ensure only privacy-preserving results are shared.
More accurate models
Minimize false-positives with precise models trained on federated real-world financial data. Achieve superior accuracy by training adaptive models on federated data and for specific contexts.
Scalable across boundaries
Securely scale across more products, sites, and geographies for insights from across your customer and partner network.
"PET-enabled collaborative analysis and learning (CAL) together with machine learning-based network analysis appears to reduce the number of false positives by up to 80% compared with the siloed rule-based method."
Project Aurora Bank for International Settlements, Innovation Hub
Unlock the value of regulated data, reduce false positives, and focus on what matters
From banks to insurances, Apheris can be used to improve fraud detection, anti-money laundering, risk scoring and much more.
Fraud detection
Anti-money laundering
Know your customer
Ready for computational access to federated data?
Get in touch for a demo and to find out how we can help you get computational access to data without data ever leaving your customer or partner’s site.